Computer vision is one aspect of Artificial Intelligence (AI) that helps to turn large volumes of image data into useful datapoints, while creating libraries of reference data for improving future searches.
Deployed on autonomous vehicles or on a server recognising uploaded images, computer vision involves the conversion of images into a machine-readable format for rapid analysis. This formatting enables the delivery of structured data at speed, and for a computer to be able to make decisions and recommendations faster than a human can.
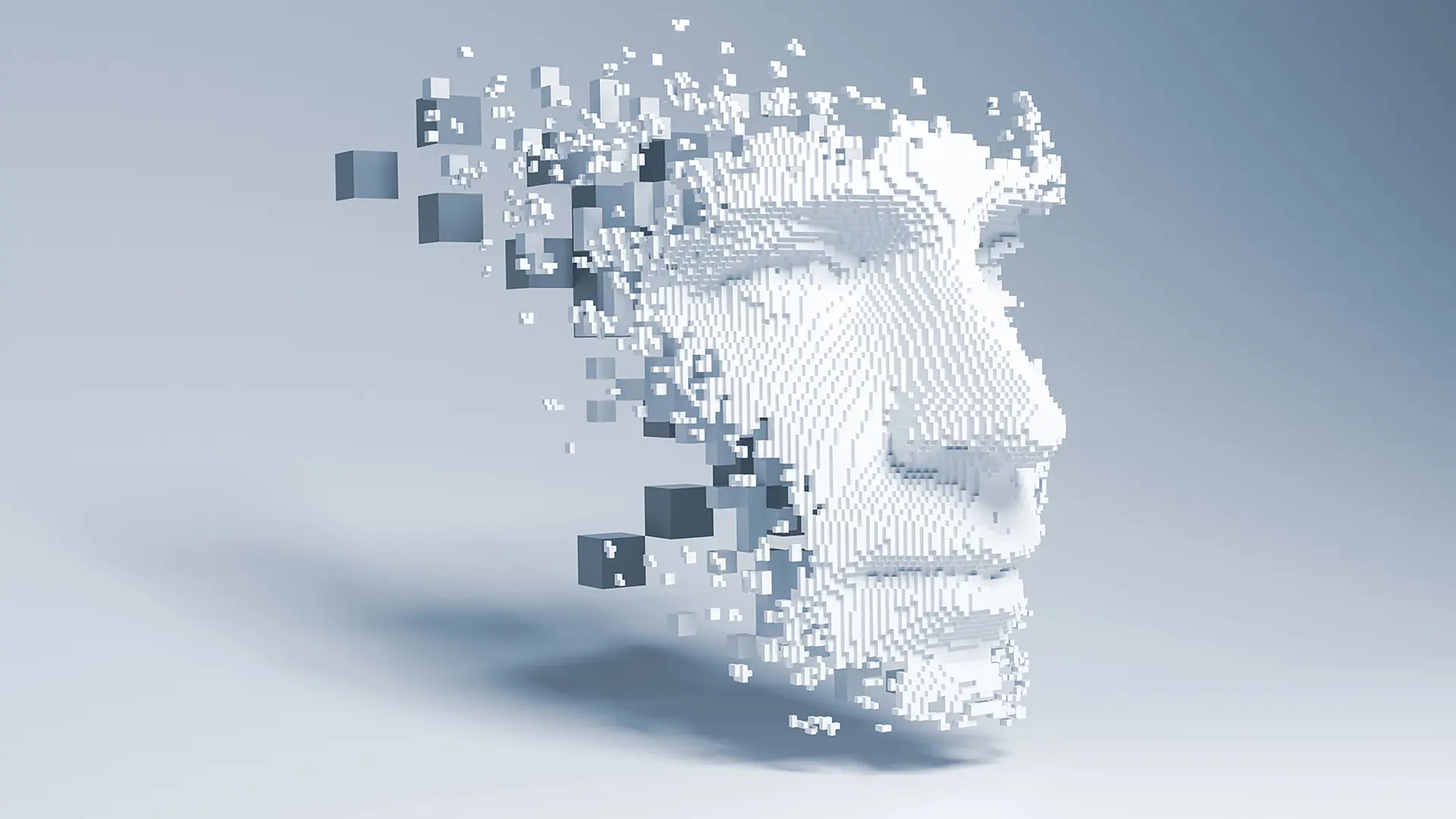
However, computer vision is the result of significant training of a process to know what to look for, and how to add value to that content. This content can involve images being classified into categories, while specific objects are further detected by the system. Being data-driven, an AI computer vision process does often rely on high levels of data quality. As a result, it can be subject to challenges such as viewpoint variation, illumination conditions, background clutter, and more.
How we work with computer vision
At Systematic we deploy computer vision in a number of ways to help with creating efficiencies in the intelligence process, thereby reducing the creation of unused or inaccessible intelligence artefacts. We use classification and object detection engines to help attach value to images, with metadata being attached to the images as they are being uploaded to the data lake, making them searchable. Being in the data lake, images can then be used to support future intelligence work based on objects, space, or time as they can be found through their metadata. This helps to reduce the cost of performing repetitive tasks, and can help add significant context to intelligence products in the future.
Image identification and classification
Two key aspects of computer vision technology involve the detection and classification of objects. Identifying an object in the first instance requires significant training of an AI system, with a detection system then drawing a box around the object to then undertake further assessment.
Classification of an object , once detected, is an additional task for an AI system to undertake. This involves the recognition of the object as something specific – such as a helicopter or military vehicle – and attaching metadata to this result. Training of an AI platform increases the accuracy of classification and metadata attribution, with the amount of training reducing over time as a library of similar images is built up through the data lake.
This information is then fed into the data lake for further storing and retrieval, as it can be used to support the understanding of enemy orders of battle, equipment dispositions, and more. This additional data can support the intelligence cycle that SitaWare Insight helps to deliver, ensuring that commanders have the latest and most comprehensive information at their fingertips.
Content-based image intelligence
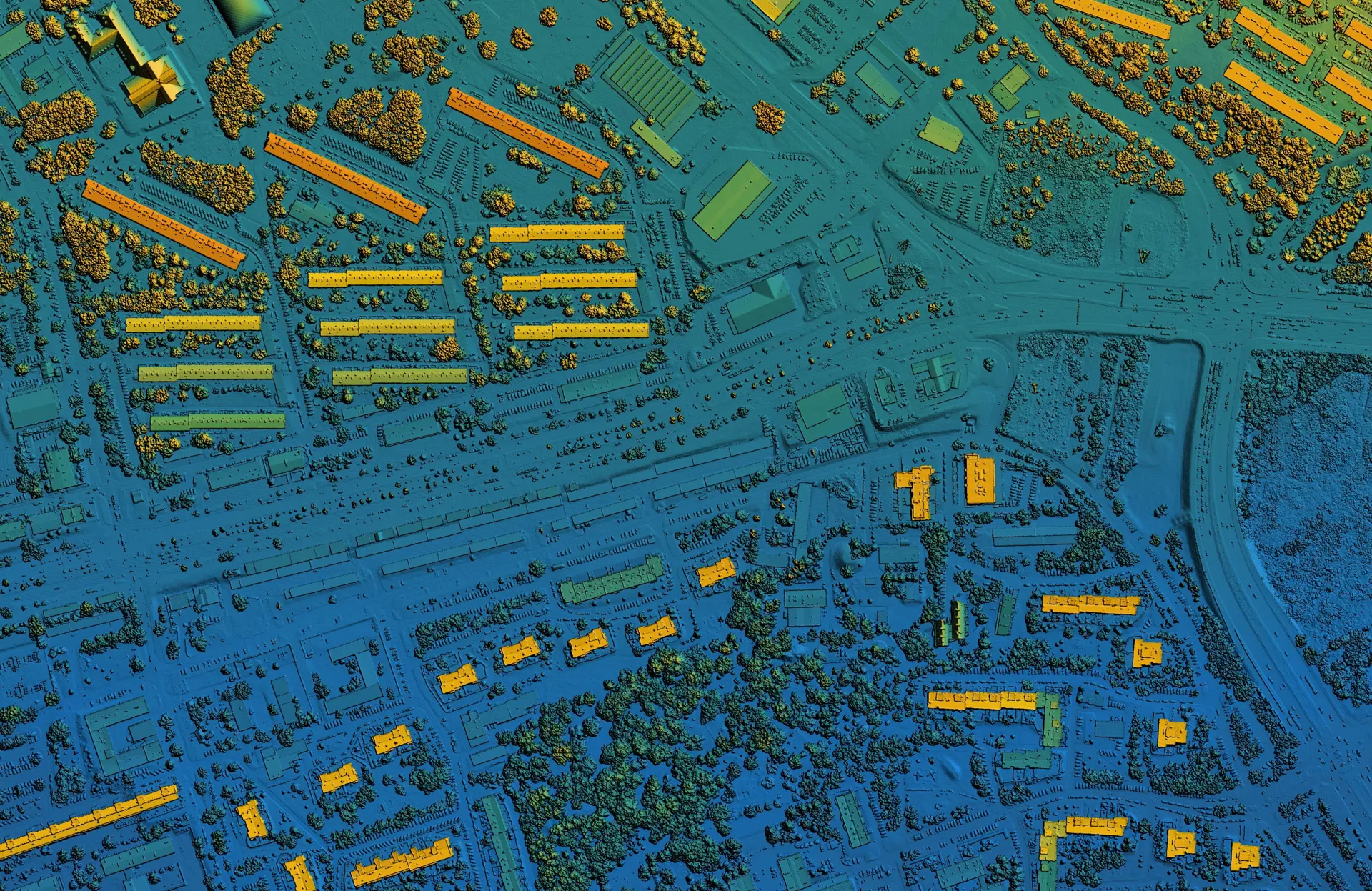
As overhead image intelligence (IMINT) has proliferated in the commercial and military domain, the need to deal with greater volumes of imagery has increased. A single location can be photographed using optical sensors, as well as space-based radar systems, airborne Lidar, infra-red photography, and more. Identifying changes across multiple areas of interest can be a time-consuming task, particularly when objects may be hard to see for a human analyst , or require greater levels of analytical expertise to interpret.
As a result of this, deploying the advanced tools of the SitaWare Insight data lake can enable interpretation of a greater range of image data types, with computer vision helping to detect, identify, classify, and tag objects as they are uploaded to the system. The anomaly detection can then be applied to identify the normal pattern of life at the location. If new types of platforms appear or numbers change significantly, a notification will be given to the user and further investigation can be done by the human operator.
This technology helps to identify new intelligence datapoints and artefacts, adding to the supporting information that can be used to deliver a commander’s critical intelligence requirements, with greater levels of transparency in the planning and decision-making process.
Explore more